Shape Retrieval with Flat Contour Segments
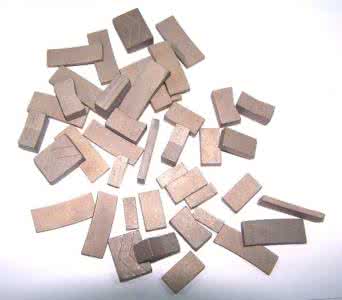
Dalong Li1, Steven Simske
Intelligent Enterprise Technologies Laboratory
HP Laboratories Palo Alto
HPL-2002-250
September 9th , 2002*
image database,
image retrieval,
run length
coding,
Freeman
coding,
down-
sampling
Content-based image retrieval (CBIR) is an important issue in the computer vision community. Both visual and textual content descriptions are employed when the user formulates queries. Shape feature is an important visual feature, as it corresponds to the region of interest in images. For retrieval, shape comparisons must be compact and accurate, and must be invariant to several geometric transformations such as translation, rotation and scaling, even if the particular representation may be rotated. In this paper, we propose a shape comparison technique based on the flat segments of the
contour. The se gmentation utilizes the Freeman coding technique and run length coding. The lengths of the flat segments make up a length vector, which are used to compare the similarity of the shapes. Experimental results from the test on the standard SQUID database are reported.
* Internal Accession Date Only 1 Georgia Institute of Technology
? Copyright Hewlett-Packard Company 2002 Approved for External Publication
Shape retrieval with flat contour
segments
Dalong Li+, Steven Simske
Intelligent Enterprise Technologies Lab
HP Laboratories Palo Alto +(Georgia Institute of Technology / HP Labs)
July 30th, 2002
ABSTRACT
Content-based image retrieval (CBIR) is an important issue in the computer vision community. Both visual and textual descriptions are employed when the user formulates his queries. Shape feature is one of the most important visual features. The shape feature is essential as it corresponds to the region of interest in images. Consequently, the shape representation is fundamental. The shape comparisons must be compact and accurate, and must own properties of invariance to several geometric transformations such as translation, rotation and scaling, though the representation itself may be variant to rotation.
In this paper, we propose a shape comparison technique based on the flat segments of the contour. The segmentation utilizes the Freeman coding and run length coding. The lengths of the flat segments make up a length vector, which are used to compare the similarity of the shapes. Experimental results from the test on the standard SQUID are reported.
Keywords: Image Database, retrieval, run length coding, Freeman Code, down-sampling. 1 Introduction
One of the issues raised by multimedia data (such as images, video, audio, graphics, text) integration in databases is the efficient retrieval of images [3]. Several prototypes [4-7] and commercial systems [1-2] have been implemented in order to address this problem. The retrieval process is based on the content, and more particularly on the visual object features.
This process requires principally two modules: the extraction and the query. The first module extracts visual features from data by using analysis techniques for each media. Then, each extracted feature is stored in the database. For example, the color feature may be represented by a color histogram [8], statistical moments [9], etc. In the second module, users formulate their query from features previously extracted. The retrieval process computes the similarity between source and target features, and sorts the most similar objects according to their similarity value. The system must be flexible since images may belong to different domains.
For images retrieval, low-level visual features are color, texture, and shape. Among these features, shape is the most important because it represents significant regions or relevant objects in images. Extensive work has been done in shape retrieval. They include tree-pruning [14], the generalized Hough transformation
[15], or pose clustering [16], geometric hashing [17], the alignment method [18], deformable template [19], relaxation labeling [20], Fourier descriptors [21], wavelet transformation [22], curvature scale space [23], neural networks [24], dynamic programming [25] and shape context [26].
In the paper, we focus on shape representation and comparisons. In section 2, we review the freeman coding and run length coding which are used in our method. Our algorithm and experiments are introduced in section 3 and section 4 respectively, followed by a conclusion.
2 Shape representation with Freeman coding
In a content-based image retrieval system, the shape matching process efficiency is essential. Consequently, a compact and reliable shape representation and a well-suited similarity distance are necessary. An interesting shape description should be invariant to translation, rotation, scaling and starting point transformations [10-11].