ThisarticlewaspublishedinanElsevierjournal.Theattachedcopy
isfurnishedtotheauthorfornon-commercialresearchandeducationuse,includingforinstructionattheauthor’sinstitution,sharingwithcolleaguesandprovidingtoinstitutionadministration.Otheruses,includingreproductionanddistribution,orsellingorlicensingcopies,orpostingtopersonal,institutionalorthirdparty
websitesareprohibited.Inmostcasesauthorsarepermittedtoposttheirversionofthearticle(e.g.inWordorTexform)totheirpersonalwebsiteorinstitutionalrepository.AuthorsrequiringfurtherinformationregardingElsevier’sarchivingandmanuscriptpoliciesare
encouragedtovisit:
http://www.elsevier.com/copyright
Author's personal copy
JournalofTheoreticalBiology247(2007)687–694
www.elsevier.com/locate/yjtbi
Predictionofproteincodingregionsbythe3-baseperiodicityanalysisof
aDNAsequence
ChangchuanYin,StephenS.-T.Yau?
DepartmentofMathematics,StatisticsandComputerScience,TheUniversityofIllinoisatChicago,M/C249,Chicago,IL60607-7045,USA
Received22October2006;receivedinrevisedform24March2007;accepted26March2007
Availableonline10April2007
Abstract
Withtheexponentialgrowthofgenomicsequences,thereisanincreasingdemandtoaccuratelyidentifyproteincodingregions(exons)fromgenomicsequences.Despitemanyprogressesbeingmadeintheidenti?cationofproteincodingregionsbycomputationalmethodsduringthelasttwodecades,theperformancesandef?cienciesofthepredictionmethodsstillneedtobeimproved.Inaddition,itisindispensabletodevelopdifferentpredictionmethodssincecombiningdifferentmethodsmaygreatlyimprovethepredictionaccuracy.Anewmethodtopredictproteincodingregionsisdevelopedinthispaperbasedonthefactthatmostofexonsequenceshavea3-baseperiodicity,whileintronsequencesdonothavethisuniquefeature.Themethodcomputesthe3-baseperiodicityandthebackgroundnoiseofthestepwiseDNAsegmentsofthetargetDNAsequencesusingnucleotidedistributionsinthethreecodonpositionsoftheDNAsequences.Exonandintronsequencescanbeidenti?edfromtrendsoftheratioofthe3-baseperiodicitytothebackgroundnoiseintheDNAsequences.Casestudiesongenesfromdifferentorganismsshowthatthismethodisaneffectiveapproachforexonprediction.r2007ElsevierLtd.Allrightsreserved.
Keywords:Exon;Intron;3-Baseperiodicity;Fouriertransform
1.Introduction
Animportantstepingenomicannotationistoidentifyproteincodingregionsofgenomicsequences,whichisachallengingproblemespeciallyinthestudyofeukaryotegenomes.Inaneukaryotegenome,proteincodingregions(exons)areusuallynotcontinuous,butare?ankedbynoncodingregions(introns).Duetothelackofobvioussequencefeaturesbetweenexonsandintrons,effectivelydistinguishingproteincodingregionsfromnoncodingregionsisachallengingprobleminbioinformatics.
Duringthelasttwodecades,avarietyofcomputationalalgorithmshavebeendevelopedtopredictexons(forreviews,FicketandTung,1992;Fickett,1996;Zhang,
etal.,2002).Mostoftheexon-?nding2002;Mathe
algorithmsarebasedonstatisticsmethods,whichusuallyusetrainingdatasetsfromknownexonandintronsequencestocomputepredictionfunctions.Asexamples,GenScan
Correspondingauthor.Tel./fax:+13129963065.
E-mailaddress:yau@uic.edu(S.S.-T.Yau).
0022-5193/$-seefrontmatterr2007ElsevierLtd.Allrightsreserved.doi:10.1016/j.jtbi.2007.03.038
algorithm(BurgeandKarlin,1997)measureddistinctstatisticsfeaturesofexonsandintronswithingenomesandemployedtheminpredictionviahiddenMarkovmodel(HMM);MZFFmethod(Zhang,1997)wasdevelopedforpredictingproteincodingregionsusingquadraticdiscrimi-nantanalysisofdifferentsequencecharactersofexonsandintrons.Ascombiningdifferentgenepredictionmethodsmayincreasetheaccuracyofthepredictiongreatly,developmentofdifferenteffectivegenepredictionalgorithmsisoneofthefundamentaleffortsingenepredictionstudy.Duringrecentyears,signalprocessingapproacheshavebeenattractingsigni?cantattentionsingenomicDNAresearchandhavebecomeincreasinglyimportanttoelucidategenomestructuresbecausetheymayidentifyhiddenperiodicitiesandfeatureswhichcannotberevealedeasilybyconventionalstatisticsmethods.AfterconvertingsymbolDNAsequencestonumericalsequences,signalprocessingtools,typically,discreteFouriertransform(DFT)orwaveletanalysis,canbeappliedtothenumericalvectorstostudythefrequencydomainofthesequences(Anastassiou,2000;WangandJohnson,2002;Kauerand
688
C.Yin,S.S.-T.Yau/JournalofTheoreticalBiology247(2007)687–694
Blocker,2003;VaidanahanandYoon,2004).Usingthesignalprocessingmethods,avarietyofgenepredictionalgorithmshavebeendeveloped(Tiwarietal.,1997;Anastassiou,2000;KotlarandLavner,2003;Jin,2004;Gaoetal.,2005).Tiwarietal.(1997)exploredthemeasureofspectralcontent(SC)inDNAsequencesbasedonthefactthatthe3-baseperiodicity,identi?edasapronouncedpeakatthefrequencyN=3oftheFourierpowerspectrumoftheDNAsequences(NisthelengthoftheDNAsequence),isprevalentinmostproteincodingregions,butdoesnotexistinnoncodingregions(Tsonisetal.,1991;Voss,1992;ChechetkinandTurygin,1995;Dodinetal.,2000).Anastassiou(2000)presentedanoptimizedSCmeasureofDNAsequencesforgeneprediction.KotlarandLavner(2003)utilizedspectralrotationmeasurebasedontheargumentsoftheDFTtodevelopanovelgenepredictionalgorithm,whichwaslaterimprovedbyJin(2004).Gaoetal.(2005)combinedthe3-baseperiodicityandthefractalfeaturesofDNAsequencestoimprovegenepredictionmethods.
MostoftheDFTbasedgene?ndingalgorithmsuseashort-sequencewindowapproach(Tiwarietal.,1997;Yanetal.,1998;Anastassiou,2000),inwhicha?xed-lengthwindowisusedtoslideaDNAsequencetocomputetheFourierpowerspectrum.However,thisapproachhaslimitations.Asmallwindowframecausesmorestatisticaloscillation,resultinginmorepredictionerrors,whereasalargewindowframemaymisssmallexonsorintrons.Thearbitrarychoicesofwindowsizemadetheshort-sequencewindowFouriertechniquesubjecttobias.Furthermore,theshort-sequencewindowFouriertransformrequiresmuchlongerCPUtime.Itbecomesachallengingproblemwhen?ndinggenesforwholegenomesasdirectcomputa-tionofFouriertransformsistimeconsuming.
Itwasdemonstratedthatthe3-baseperiodicityinaDNAsequenceispartlycausedbytheunbalancednucleotidedistributionsinthethreecodingpositionsinthesequence(Fickett,1982;FicketandTung,1992;Tiwarietal.,1997;YinandYau,2005).Inanexonsequence,thenucleotidedistributioninthethreecodonpositionsisunbalanced,whileinanintronsequence,thenucleotidesdistributeuniformlyinthethreecodonpositions.Thereasonoftheunbalanceddistributionisthatproteinspreferspecialaminoacidcompositionsandthusnucleotideusageinacodingregionishighlybiased(FicketandTung,1992;Tiwarietal.,1997;YinandYau,2005).Thispaperpresentsanextensionofthecurrentgenepredictionalgorithms(Tiwarietal.,1997;Anastassiou,2000),calledEPNDmethod(exonpredictionvianucleotidedistribu-tions),whichisbasedonthepeakatthefrequencyofN=3oftheDFTandthefrequenciesofthenucleotidesinthethreecodonpositions(positionasymmetrymeasure)withinknowngenes.Thealgorithmistestedforidentifyingexons/intronswithinknowngenesfromseveralorganismsinthispaper.Casestudiesindicatethatthemethoddescribedinthispaperisaneffectiveproteincodingregionpredictionmethodintermsofaccuracyandef?ciency.
2.Methodsandalgorithms
2.1.FourierspectrumanalysisofDNAsequences
AsymbolicDNAsequence,denotedas,xe0T;xe1T;...;xeNà1T,is?rstconvertedtofourbinaryindicatorsequences,uAenT;uTenT;uCenT,anduGenT,whichindicatethepresenceorabsenceoffournucleotides,A,T,C,andG,atthenthposition,respectively(Voss,1992;Tiwarietal.,1997;Anastassiou,2000).Forinstance,theindicatorsequence,uAenT?0001010111...;indicatesthatthenucleotideAisinthepositions4,6,8,9,and10oftheDNAsequence.
TheDFTconvertsasignalinthesignaldomaintoasetofnewvaluesinthefrequencydomain.ForasignaloflengthN,fenT;n?0;1;...;Nà1,itsDFTisde?nedasfollows:NFekT?
Xà1fenTeài
2pnk
(2.1)
n?0
wherei?p??????à1?
.TheDFTpowerspectrumofasignalatthefrequencykisde?nedas:PSekT?jFekTj2;
k?0;1;2;...;N,
(2.2)
whereFekTisthekthDFTcoef?cient.
TheDFTpowerspectrumofaDNAsequenceisthesumofthepowerspectrumofitsfourbinaryindicatorsequences(SilvermanandLinsker,1986;Tiwarietal.,1997;Anastassiou,2000):
PSekT?PSAekTtPSTekTtPSCekTtPSGekT
(2.3)
wherePSAekT;PSTekT;PSCekTandPSGekTaretheFourierpowerspectrumofthefourindicatorsequencesuAenT;uTenT;uCenTanduGenT,respectively.DuetothesymmetrypropertyoftheDFTspectrumofrealnumbersignals,the?guresinthispaperonlyshowhalfoftheFourierspectrumofDNAsequences.
2.2.Computingthe3-baseperiodicityandbackgroundnoisefromnucleotidedistributionsofaDNAsequence
TheasymmetryinthenucleotidedistributionsinthethreecodonpositionsanditsconnectiontotheDFTpeakinN=3atcodingregionswereaddressedbyFicket(Fickett,1982;FicketandTung,1992).The3-baseperiodicitymagnitudeandbackgroundnoisecanbedirectlycomputedfromthenucleotidedistributions(FicketandTung,1992;YinandYau,2005).LetFx1;Fx2;Fx3betheoccurrencefrequenciesofthenucleotidex2fA;T;C;Gginthe?rst,thesecondandthethirdcodonpositions,respectively.Thenthe3-baseperiodicitymagnitudecanbecomputedasfollows:
PSeN=3T?X
?F2x1tF2x2tF2
x3
x?A;T;C;G
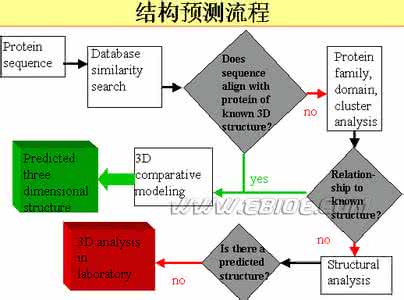
àeFx1?Fx2tFx1?Fx3tFx2?Fx3T??.
e2:4T
C.Yin,S.S.-T.Yau/JournalofTheoreticalBiology247(2007)687–694
689
ThebackgroundnoiseofaDNAsequenceoflengthN,representedastheaveragepowerspectrumEoverallthefrequencies,isdeterminedmainlybythelengthoftheDNAsequence(YinandYau,2005).Thus,theratioofthe3-baseperiodicitysignaltothebackgroundnoiseofaDNAsequence,denotedasSNeNT,isde?nedasfollows:SNeNT?PSeN=3T
N
.(2.5)
SNeNTcanbeinterpretedasstrengthofthe3-baseperiodicitypernucleotide.Basedonthecomputationalsimulationofcomputergeneratedsequencesandveri?edwith12exons/intronsfromYeastandC.elegans,itwasshownthatSNeNTisequaltoorlargerthan2formostexonsequences(YinandYau,2005,alsorefertoFig.3),whileitislessthan2formostintronsequences.Thethresholdvalueofthesignal-to-noiseissetto2inthegene?ndingalgorithminthispaper.
2.3.Algorithmforexonpredictionbynucleotidedistribution(EPND)
ForaDNAsequenceoflengthN,letDkdenotetheDNAwalksequenceoflengthk,i.e.,Dkisthesub-regionoftheDNAsequencerangingfromthebeginningtothepositionk.To?ndexonregionsandintronregionswithinthegivenDNAsequence,theEPNDalgorithmisdevel-opedasfollows,andthe?owchartofthealgorithmbelowisshowninFig.1.1.Setk?1.
2.ComputenucleotidedistributionsofDkinthethreecodonpositionsofFxi(x2fA;T;C;Gg,i2f1;2;3gT.ThenucleotidedistributionofaDNAwalksequenceoflengthkcanbeobtainedrecursivelyfromtheDNAwalksequenceoflengthkà1withtheoccurringfrequenciesofthenucleotidesonthepositionk.
3.Computethemagnitudeofthe3-baseperiodicityPSek=3TinDkbasedontheformula(2.4).
4.Computetheratioof3-baseperiodicitytobackgroundnoise,SNekT?PSek=3T=k,withintheDNAsequenceDk.5.Increasekby1andrepeatstep2tostep4,untilk?N.
6.ComputetheslopeofSNateachpositionontheSNplotasfollows:sincemostoftheexonorintronsequencesinagenomearelongerthan50basepairs,theslopeattheithpositioniscomputedaseSNeiTàSNeià50TT=50,whereiisfrom51toN.
7.Setthenucleotideateachpositiontoexonorintronregionasfollows:iftheslopeatthepositionislargerthan0andSNislargerthanorequalto2,setthenucleotideatthepositionasexonnucleotide;otherwise,setitasintronnucleotide.
8.Reducelocalnoise.IfaDNAregionlessthan50basepairsisidenti?edasanintronfromstep7,andis?ankedbytwoexonregions,thisregionisoftenafalsenegative,andisresetasexonregion;similarly,ifaDNAregionlessthan50basepairsisidenti?edasanexonfromstep7,andis
?ankedbytwointronregions,thisregionisoftenafalsepositive,andisresetasanintronregion.
2.4.Improvingpredictionaccuracyusingdifferentstartingpoints
ForalongDNAsequencethatmaycontainmorethantwoexons(ortwointrons),suchasexon–intron–exon,theaccumulatedsignal-to-noiseratioofthelastexonwillbecomelowespeciallywhentheintroninbetweenislong,whichmayaffecttheaccuracyoftheprediction.ItwouldimprovethealgorithmifwedivideaDNAsequenceintodifferentsubregions.Inaddition,toreducefalseexonsandfalseintrons,weapplythealgorithmatdifferentarbitrarystartingpointssothateachnucleotidemaybetestedmultipletimes.ThefollowingalgorithmisdevelopedtoimproveexonpredictionaccuracywhenusingEPNDmethod:
1.IfaDNAsequenceislongerthan2000basepairs(bp),divideittosub-sequencesof2000basepairs.
2.Foreach2000basepairssub-sequence,setP1?1;P2?401;P3?801;P4?1201;P5?1601andP6?2000bethesixeven-spacedpoints.
3.IdentifyexonorintronnucleotidesusingEPNDmethodonthesub-sequencebetweenpointPiandP6wherei?1;2;3;4;5.SoeachnucleotideafterpointsP3istestedatleastthreetimesusingEPNDmethodfromdifferentstartpoints.Anucleotideisidenti?edasanexonnucleotidewhenitispredicatedinanexonregioninthemajorityofthetests.
2.5.DatabaseandmeasuresforperformanceevaluationThedatasetusedfortheevaluationoftheperformanceoftheEPNDmethodisXpro(Gopalanetal.,2004),whichcontainstheeukaryoticproteincodingDNAsequencesfromGeneBankrelease139.ThedatasetwasdownloadedfromtheXprowebsiteas?at?les(Xproversion:v.1.2,2004,http://origin.bic.nus.edu.sg/xpro).One?le,exonse—intron—139.gz,containsproteincodingregions(exons),andtheother?le,intronseq—intron—139.gzcontainsnon-proteincodingregions(introns).Both?lesconsistofDNAsequencesandthecorrespondingheaderinfor-mationwhichindicatesgenelocus,organismthatthegenesbelongto,intronlengthsandtheircorrespondingpositionswithinthegenes.Basedontheintronpositionsintheheadersections,intronsareconjugatedwiththecorre-spondingexonstoformafulloriginalgenestructurebeginningwithastartcodonandendingwithastopcodon.Thefulllengthgenesareusedinthisstudytotestalgorithmperformance.
TheperformanceoftheEPNDalgorithmismeasuredintermsofsensitivity,speci?cityandaccuracy,whicharede?nedintheliteratureasfollows(BursetandGuigo,1996).Thesensitivity,Sn?TP=eTPtFNT,andthespeci?city,Sp?TN=eTNtFPT,whereTPisthetruepositive,whichisthelengthofnucleotidesofcorrectly
百度搜索“爱华网”,专业资料、生活学习,尽在爱华网!